Portfolio Optimization
Worst-case scenario optimization
The traditional portfolio optimization framework assumes that parameters of the analytical model are known precisely. In practice, however, statistical estimates of parameters may have significant estimation error. In particular it is relevant for the sample estimate of vector that represents the excess part of expected instantaneous rates of return. Ignorance of estimation error in usually leads to unwarrantably extreme values of portfolio weights and dramatic shifts in portfolio structure when previous estimates are modified with recent historical data.
One way to avoid the mentioned above undesirable properties of an optimal portfolio is to include the estimation error explicitly in optimization process. Most practically sound results in this direction were obtained in [Garlappi, Uppal, Wang; 2005]. The authors implement the following algorithm, which they call Multi-Prior Approach:
- The set
of possible values for the true vector is defined.
- The optimal portfolio, corresponding to relative risk aversion coefficient
, is obtained by solving the following Min-Max problem:
Note. To consider the robust version of the target shortfall probability criterion the above Min-Max problem must be modified in the following way:

In other words, the investor seeks for portfolio with the best performance under the worst-case scenario. Inner minimization over expected instantaneous excess returns in the above expressions can be regarded as reflection of investor’s uncertainty aversion. A convenient measure for uncertainty aversion degree is confidence level , used below to define . As approaches 1, investor’s aversion to uncertainty increases to infinity.
Two versions of set are considered below.
Separate Confidence Intervals
Let be the number of portfolio components and be sample counterpart of . For every corresponding confidence interval for in the form of is obtained by solving the following equation:

The above equation has the following solution:
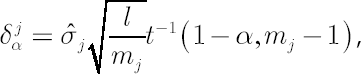
where
– sample volatility in -th asset
– average number of observations in one year
– available number of observations in -th asset
– inverse function of Student’s t-distribution with degrees of freedom: it returns value such that , where is -distributed random variable with degrees of freedom.
Set , corresponding to Separate Confidence Intervals for , is defined as -dimensional rectangular parallelepiped .
Then, the stated Min-Max problem, corresponding to relative risk aversion coefficient , reduces to

where portfolio worst-case excess Mu is defined in the following way:
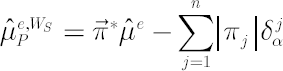
Analogously, the Min-Max problem, corresponding to the target shortfall probability criterion, simplifies to following:

In case of uncorrelated assets the probability of falling into is . In the general case calculation of the probability of falling into is more complicated. It could be desirable to explicitly state the probability of falling into than defining it implicitly by means of confidence intervals for individual assets. This is done in the next section.
Joint Confidence Interval
Suppose all portfolio components possess the same number of observations . Consider the quantity , which is a sample estimate of squared instantaneous Sharpe ratio for tangency portfolio, corresponding to portfolio excess Mu equal to . This quantity is a convenient measure for the distance between and .
Joint Confidence Interval for is defined in the following way:

Solution for has the following form:

where is an inverse function of -distribution with and degrees of freedom: it returns value such that , where is -distributed random variable with and degrees of freedom.
Set , corresponding to the above joint confidence interval for , is defined as follows:

The Min-Max problem, corresponding to risk aversion coefficient , simplifies to

where portfolio worst-case excess Mu is defined as 
Correspondingly, the Min-Max problem, corresponding to the target shortfall probability criterion, simplifies to the following:

| |
|